Offer Types
Targeted and personalized offers have much higher levels of engagement versus anonymous ‘spray-and-pray’ incentives. Targeted and personalized offers are closely related but they are not necessarily the same. If a digital promotion targets consumers only with specific demographic characteristics, it is considered a targeted offer. If incremental purchase data, from customers within the same demographic profile, is incorporated into the coupon selection process; it provides the opportunity to deliver a personalized offer.
Value Creation
Regardless of the digital distribution channel, the ability to leverage shopper data enables the delivery of more relevant and compelling real-time offers to the intended audience. These data sources might include past purchase history, preferred retailer formats or brand relationship parameters, to name but a few. Targeted and personalized offers inevitably enhance the customer experience bringing the brand and customer closer together.
Behavioral Attributes
Targeted offers are significantly more effective than anonymous offers, yet many targeted offers ‘miss the mark’ as the offer is not personalized. Personalizing the offer, a step beyond simple targeting, results in a much higher customer engagement and a better shopper experience.
Every shopper, who has visited an online shopping website, will see subsequent advertisements – featuring offers – on other websites related to the products they were initially browsing. This is an example of simple retargeting, based only on browser history passed through the URL. The problem with these ‘retargeted’ advertisements is that only one piece of data is used to determine the offer, in most cases. While the advertisement, and featured offer, is targeted it might still be less effective because it lacks personalization.
Consider a consumer shopping online for a snow blower. Based on their browsing history, it is seems reasonable to display advertisements for snowblowers on other visited websites, using a third-party retargeting vendor. Reasonable does not imply efficient. Neglecting the inclusion of purchase data suggests displaying retargeted advertisements for snowblowers to consumers that might have already made that purchase. With purchase data, the consumer could be retargeted with advertisements for adjacent products such as winter clothing.
Actionable Data
Leveraging all available first-party, second-party and third-party customer data provides an ideal opportunity to personalize the offer and makes the targeting much more precise.
Omnichannel Engagement Parameters
A comprehensive solution will integrate all customer engagement data, both online and offline, to influence the selection of the best product offer. The actual identity of the consumer need not be known. Presentation of the offer needs to be dynamic and should always adapt to what is known about the consumer. Matching purchase transaction data, and other engagement parameters, allows for a compelling cost-effective presentation of an upsell or follow-on offer or an adjacent product offer while the original purchase is still fresh in his or her mind.
Rules-Based Engine
Independent of the distribution channel personalization opportunities exist for new and existing customers.
A paid search campaign for glass cleaner is a good metaphor. Shoppers might be searching against a broad variety of keywords that match the specific campaign criteria. Depending on the search term(s), the offer can be better personalized to match the shopping behavior of the specific individual. Consumers searching generic “glass cleaner” implies they are not brand loyalists and may require a higher value offer to consider purchasing the product. Conversely, the shoppers who search for a specific brand of glass cleaner indicate a higher level of purchase intent, possibly a brand loyalist or someone with strong brand affinity. This scenario warrants the consideration of an offer for the purchase of two bottles or a jumbo size bottle given the brand choice has arguably been made.
Anonymous shoppers may still be identifiable by a cookie or other connected identifiers. The ability to link consumer activity provides for another level of personalization. This knowledge might yield a decision to deliver a higher value offer that is more compelling. Conversely, it might be determined that the shopper has already engaged with this offer, and has exceed the number of offers it is entitled to during a specified time period and therefore should receive an alternative or adjacent product offer.
For known audience segments, such as email lists or website registrants, consumer behavioral data is very powerful in enhancing personalization. Ambient content should be dynamically adjusted for the individual consumer in the same way as the coupon offer. Past purchase history might highlight that the shopper has frequently purchased at a specific retailer so a complimentary offer at that retailer may be just the thing to catch his or her attention.
Promotion Strategy
Don’t allow seemingly insurmountable barriers prevent to the implementation of a personalized digital promotions strategy. Use all relevant data advantageously and avoid collecting irrelevant data ‘for the sake of collecting it’. Personalized offers are far more accurate and ultimately more engaging, two critical variables that define the success of a promotional program.
Practical Results
For shoppers who print the coupon offers, the conversion or redemption rates are 48% for targeted promotional offers and as high as 82% for personalized offers.
About the Author
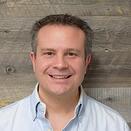
GREG HANSEN
Chief Technology Officer & Co-Founder
Greg has more than 20 years of technology experience, and has designed, developed, and implemented RevTrax’s core technology and systems. He leads the company’s information technology team as Chief Architect and Systems Administrator. Before joining RevTrax, Greg was the Chief Application Architect for Diversified Investment Advisors. Greg has a proven track record of bridging business requirements with technology-driven solutions. He holds various patents for navigation algorithms and has extensive experience with design patterns, data structures and enterprise architecture. Working in a collaborative fashion with business partners, Greg has lead large integration efforts and served as an advisor for the technical teams at numerous organizations. Greg received his Bachelor’s degree in Computer Science from Stevens Institute of Technology.